For every news report about the latest breakthrough in artificial intelligence (AI), we also see plenty of stories about AI failures in the business world. One study estimates that 70 percent of AI initiatives “see no or minimal impact,” putting the blame on a variety of factors: lack of expertise, misunderstanding of AI capabilities and under budgeting, among other issues.
As we developed our SoftwareOne Intelligence Fabric methodology for successful AI implementation, we also identified many common stumbling blocks. By staying alert to these kinds of issues, you can avoid the AI project failures that have cost many other organisations far too much time, money and energy:
Unclear strategy
Imagine a retailer that wants to provide more personalised product recommendations to its online customers while also improving its inventory management. Its goals are to enhance the customer shopping experience, increase sales and reduce the costs of both overstocking and understocking. So it develops predictive modelling for inventory and integrates AI recommendation algorithms into its e-commerce platform – only to see both solutions fail to deliver on expectations.
What went wrong? The company’s biggest misstep happened early in the project, when it didn’t invest the time and effort needed to clearly identify its desired business outcomes and ensure that its AI programme aligned strategically with its business goals. As a result, the project ended up focusing on technology rather than on the specific problems that the company wanted to solve – which led to underwhelming results.
Poor user experience
In this case, a healthcare provider saw AI as a way to automate patient appointment scheduling and improve patient care through personalised follow-up interactions. By doing this, it aimed to increase patient satisfaction and operational efficiency while also optimising workloads for its busy staff. But the organisation made the mistake of not paying attention to what the AI experience would be like for its end users.
Soon after the solution rolled out, patients were complaining. Using the chatbots was cumbersome, they reported, and interactions were impersonal and unsatisfying. So chatbot adoption remained low, leaving the company short of its hoped-for benefits. The organisation could have prevented this outcome with a greater focus on user-centric design. This would have enabled it to customise its large language models (LLMs) to be more empathetic, engaging and user-friendly.
Weak MLOps
Predictive maintenance promised to help a manufacturer minimise downtime, improve product quality and optimise its costs for maintaining factory equipment. But the AI algorithms that the company implemented didn’t deliver the real-time maintenance advice and other benefits it expected.
The problem came down to ineffective machine learning operations (MLOps), a practice that builds on successes of DevOps to support continuous integration and development in AI applications. With its algorithms built on a poor foundation, the manufacturer’s data analyses and predictions ended up being highly inaccurate. Had it started with stronger MLOps, the organisation could have more easily built, monitored and updated machine learning models that reflected reality.
Inadequate data foundations
Looking to improve security and customer satisfaction, a financial services business hoped to use AI to detect fraudulent activities. But it failed to build the right foundation by properly integrating data from across its systems. As a result, the algorithms it implemented didn’t work effectively to identify fraud and suspicious transactions.
Implementing AI successfully requires getting data in order first. That means not only ensuring that the data used to build a solution is accurate and reliable, but that information is clean, complete and usable for the intended purposes.
Lack of adoption support
A telecommunications company saw an opportunity to improve customer satisfaction and reduce costs by introducing AI-powered chatbots to handle common customer queries. It trained LLMs using its own historical customer interaction data with the goal of providing more efficient and targeted responses to customer questions. But the new solution met with resistance from both employees and customers.
Although the AI solution itself performed according to expectations, the company failed to pave the way for adoption by end users. Employees lacked the training needed to use the technology effectively, and customers had no warning or input before the new system was put in place. The telco could have better managed the change through proactive communication with all users, proper training for employees and a phased rollout that allowed for refinements based on customer feedback. It would have also benefited from more user-friendly support structures such as FAQs and help desks.
Data and business strategies for successful AI initiatives
Wiktor Zdzienicki, Global Data & AI Practice Manager at SoftwareOne, dives deep into what it truly takes to make AI work:
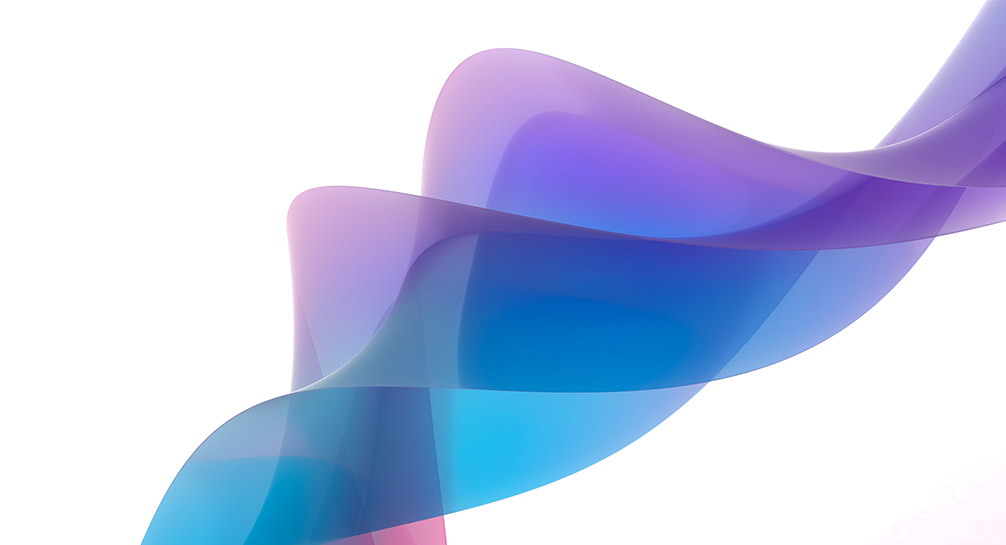
These are just a few examples of potential stumbling blocks when implementing an AI initiative. SoftwareOne can help you avoid costly mistakes and missed opportunities. SoftwareOne Intelligence Fabric is our suite of accelerators, practices, and assets to help businesses establish a strong foundation, reduce risk, and obtain repeatable industry patterns to succeed with AI.
Learn more about what AI can do for you
SoftwareOne demystifies AI and helps your team understand the value and risks, pragmatically defining the capabilities needed for your organisation to adopt data-driven practices and scale analytics and AI.
Reach out today to schedule a free 1-hour workshop for you and your team.
Learn more about what AI can do for you
SoftwareOne demystifies AI and helps your team understand the value and risks, pragmatically defining the capabilities needed for your organisation to adopt data-driven practices and scale analytics and AI.
Reach out today to schedule a free 1-hour workshop for you and your team.